Valorem's Global R&I Director shares edge computing trends that are enabling advanced insights through Edge AI Video Analytics.
Artificial Intelligence (AI) is an ongoing trend with continued growth disrupting almost every aspect of our lives. Not just in the professional world with Industrial IoT (IIoT), robotics, automation and more but also use cases that are becoming a seamless and unobtrusive part of our day-to-day life. This trend is fueled by edge computing which is providing opportunities to move AI workloads from the Intelligent Cloud to the Intelligent Edge for improved response times and bandwidth savings. The benefit of edge deployments is especially strong when it comes to computer vision models that take large data streams like images or live video as input. With edge computing, these large data streams can now be processed locally at the device/end-user level eliminating the need for significant bandwidth or privacy risks associated with streaming into a cloud data center. Edge video analytics systems can execute computer vision, deep-learning algorithms either directly integrated into the camera or with an attached edge computing system. This does not mean there is no need for the cloud anymore. Not at all. A hybrid approach with triggered business processes and further cloud execution is typically the best approach. Leveraged computer vision AI models are usually pre-trained in the cloud and are then deployed to an edge device. This approach is using the best of both worlds where the power and scalability of the Cloud is used during the resource intensive training phase and the short latency of the Edge is used during real-time model inference / evaluation.
We mentioned Edge Computing and Analytics in our Top Trends for 2021 post as a technology to watch for significant growth this year. In this post, we will share some more Edge AI market research encouraging business leaders to begin integrating this cutting-edge tech in their business processes to prepare for changing customer and employee needs. In addition, we will share examples of Edge AI video analytics solutions in market today and introduce our own workplace safety Edge AI solution.
Analysts Predict Increased Shift to Edge AI for Video AnalyticsMarket research company Gartner is categorizing Edge Video Analytics in an adolescent maturity phase with a transformational character, which is Gartner’s highest benefit rating. They see the drastic impact automating real-time analytics and decision making could have compared to the already widely used camera systems where the data is often stored and analyzed later, mostly involving human labor. Having video analytics within edge systems will enable a wider range of scenarios with higher efficiency such as:
- Real-Time Traffic Monitoring and Dynamic Situational Adaption
- Facility Inspection Systems
- Recognition-Based Security Systems
- Workplace Safety Solutions
Additionally, autonomous mobile robots (AMRs) and in general, autonomous vehicles, rely on real-time object detection and the ability to segment and interpret the surrounding physical environment. Mobile robotics go hand in hand with IoT and Edge AI as they are basically intelligent IoT Edge devices on the move. To learn more about AMR, IoT and Mixed Reality, you should read this earlier post and watch our Ignite 2021 session.
Live Video Analytics with Azure
IoT is a growing trend for many businesses seeking to innovate and optimize through the cloud and the edge. IoT Edge from Microsoft supports a wide range of edge services and various AI workloads with one particularly interesting new computer vision offering called Azure Video Analyzer, previously known as Live Video Analytics on IoT Edge. With the Azure Video Analyzer platform, users can run real-time AI video analytics on the edge and can also plug-in to existing video management systems (VMS) like CCTV and similar. Also, analytics can be processed on the edge or in combination with cloud services to adapt to the enterprise needs and incorporate cloud business logic. Azure Video Analyzer functionality can be combined with other Azure IoT Edge modules such as Stream Analytics on IoT Edge, Cognitive Services on IoT Edge and Azure services in the cloud such as Event Hub, Cognitive Services, etc. to build powerful edge and hybrid applications. This has many use cases, including COVID-19 resilience which we covered here and many other return-to-work or workplace safety scenarios just to name a few.
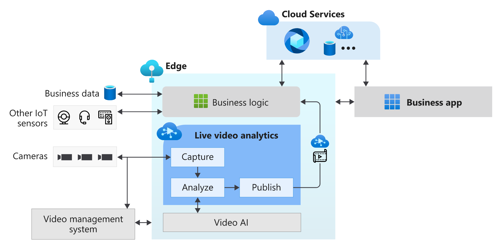
Another exciting new Edge AI offering that was announced at Microsoft Ignite 2021 is Azure Percept. Azure Percept is basically an end-to-end solution to easily build intelligent edge deployments with software services AND prebuilt development kits called Azure Percept DK. This allows developers to get started without having to solder or assemble a custom IoT sensor solution. The DK is built in a modular fashion with different modules for vision but also audio workloads. It also ships with a collection of pre-built AI models, like object, anomaly detection or keyword, sound perception. Of course, it is also possible to build custom models nicely streamlined with the Azure Percept Studio application. Including the semi-automatic generation of Deep Learning training data with automatic triggered captures. And Azure Percept is not just for pure development and R&D tasks, but can directly scale into production. The devices can be pre-configured in Azure Percept Studio and once turned on, they will automatically connect to the network and be available right away with the correct configuration thanks to the support of Wi-Fi Easy Connect.
Azure Percept is quite amazing as it provides ready-to-use dev kits with an accelerated time to ship and reduced complexity. One doesn't need to build all these small details themselves but instead can focus on the domain specific topics. You can learn more details about Azure Percept in my Ignite 2021 recap video here.
Increasing Workplace Safety with Edge AIMicrosoft’s largest developer conference Build recently took place and introduced some great new developer tooling and services. There were many great sessions. One of my personal highlights was Scott Hanselman’s keynote which featured guest speakers including Microsoft CTO Kevin Scott. Kevin’s keynote segment showed the great AI research and impact of Microsoft’s innovation. This time Kevin also introduced a new device kit which Adafruit developed in partnership with Microsoft’s Lobe.ai platform. The Adafruit ML Kit for Lobe allows you to quickly create custom low-cost Edge AI solutions that directly run an advanced Raspberry Pi 4 board. In combination with Microsoft’s low-code AI platform Lobe.ai , custom AI vision models can be created rather fast and deployed on the Raspberry Pi for a quick iterative Edge AI development approach.
In the below video I did exactly that and created a custom image classification Deep Learning model that can detect if the person in front of the camera is wearing “Safety Glasses” or “No Glasses”. If the person is not wearing safety glasses the 3 LEDs on the device will turn red. If safety glasses are worn, a green light is shown. I also trained a 3rd classification class called “Glasses” which is for normal prescription glasses. I did this to challenge the Lobe.ai vision model as it is quite hard to distinguish between normal and safety glasses. I trained the model with over a hundred images for each of the 3 classes under various lighting conditions also while I was wearing different outfits. I also tested it with other people and the AI model generalized well enough to not just work with me and it can in fact also distinguish between safety and normal glasses, which is quite impressive.
The whole AI inference / evaluation is running directly on the Raspberry Pi providing a custom Intelligent Edge AI solution for low-cost in a small standalone form factor. This solution does not require additional hardware nor internet connectivity and does not send any camera data into the cloud. A hybrid approach with a combination of edge and cloud workloads is likely the best solution to trigger certain cloud workflows, provide additional analytics with Power BI, and other integration with business processes.
It is worth mentioning that this solution runs near real-time and could be further enhanced to provide higher performance using a board with a dedicated GPU or specialized AI accelerator chips, like TPU or VPU like an Intel Movidius, NVIDIA Jetson Nano and others available in the market. Regardless of which edge device is used, it is an exciting time where we can quickly build custom intelligent Edge AI solutions with very little coding involved. In one of the future posts, we will dive a bit deeper into the rapidly growing world of low-code and no-code AI tools and services.
Are you ready to build your own customized Intelligent Edge AI solutions with us or want to learn more? Valorem Reply’s Data & AI team can help you to reach your goals quickly and efficiently. Reach out to us as marketing@valorem.com to schedule time with one of our industry experts.