There are several factors driving the interest in predictive analytics. It is a unique time in history where the confluence of several loosely correlated changes are occurring that drive the corporate passion (or should I say necessity) for machine learning and predictive analytics.
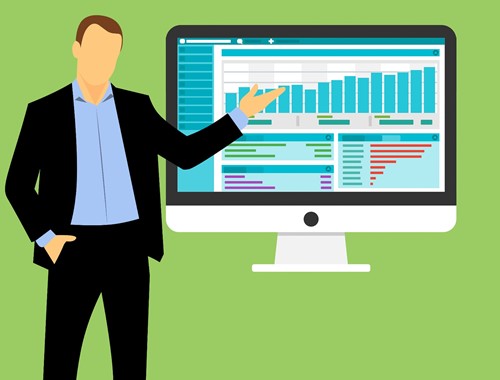
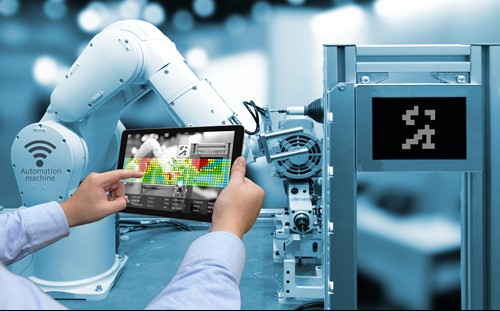
- In the 1700’s mechanization, the steam engine, the weaving loom and iron production introduced factory systems that replaced manual production.
- In the 1800’s, electrical energy, telegraph lines, mass production and the assembly line readily accelerated factory production systems. Output multiplied many times.
- A century later introduced the digital revolution. This revolution was driven by the rise of digital computers and the internet. As these technologies became widespread, it enabled information to be generated and shared faster and more easily, opening up new possibilities for economic, social, and technological innovation.
- Today marks the beginning of the 4th Industrial Revolution. We are in our infancy leveraging data to learn and automate many processes in a way that significantly accelerates decision making. The rise of predictive and prescriptive analytics, IoT and AI analytics disrupts every decision in every business.
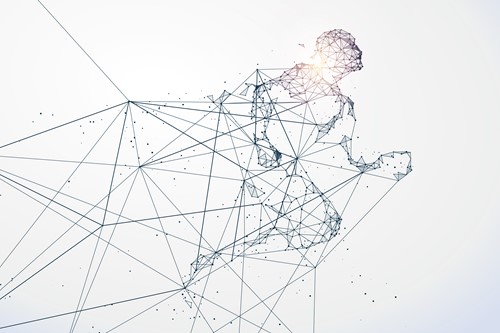
- A hundred years ago, the average lifespan of a company listed on the S&P 500 was 67 years. Today, that average lifespan of a company on the S&P 500 has decreased to just 15 years. It is becoming harder for companies to stay in the lead, or even in business, for very long.
- Richard Foster, a Yale University professor and former McKinsey partner, predicts that in the next decade, only 25% of the companies currently listed on the S&P 500 will remain there, meaning the other 75% will be replaced by new companies. These new companies will be those that take advantage of innovative technological capabilities to rapidly gain ground in their chosen markets. Similarly, the existing companies that remain on the index will be those that rapidly innovate and evolve their businesses.
- In their book, The Second Machine Age, MIT economists, Eric Brynjolfsson and Andrew McAfee propose that big data, computation, and innovation are changing our economy and institutions with a magnitude greater than almost anything ever seen in history.
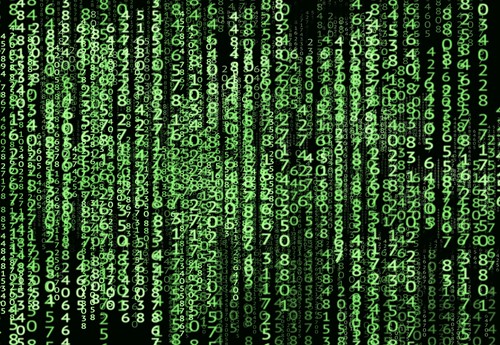
We’ve got data everywhere. It is being created faster than ever. We need to use this to keep up with the accelerating rate of change or we risk becoming irrelevant. Businesses stand to gain tremendous value from this trove of digital material, but doing so can be overwhelming. These datasets are vast, varied, and ever mutating. In order to derive value from these assets, businesses need advanced tools capable of quickly parsing out relevant information and making connections across disparate data sources, both inside and outside of the enterprise. How do we do this? There is only one answer: Machine Learning and AI. For both of these solutions, data is key – data is the new currency.